Developing Weather Control Method to Induce Meteorological Conditions
for Mitigating Heavy Rainfall Over Land
There are three hurdles in manipulating weather. First, we need to find effective interventions. Careful selection of interventions is necessary, as an ill-planned intervention may not necessarily produce the desired outcome. Secondly, interventions must be feasible. Weather, being large-scale, can require enormous amounts of energy to control, while there are also constraints on the amount of energy humans can expand. Finally, the interventions must be computable. Overcoming the large-scale nature of weather phenomena, interventions must be computed in the short time between when heavy rainfall damage is predicted and when it occurs. Although the control theory can be essential to overcome these three hurdles, the current control theory has not dealt with such large-scale phenomena.
To clear these hurdles, two research tasks have been established in our item. Item 1-1 “Development of Feedback Control Theory” develops the concept of feedback control and constructs a control theory that can overcome the above hurdles. To complement this subject, Item 1-2 “Development of Data-driven Control Theory” develops weather control methods that transcend the framework of existing control theory by utilizing data technologies such as ensemble prediction in addition to AI techniques. Through the promotion of these subjects, our item will demonstrate the feasibility of mitigating heavy rainfall over land through offshore rainfall generation on a computational basis.
Item 1-1Development of feedback control theory
Principal investigator: Toshiyuki Ohtsuka
Outline
This study starts to research a feedforward control method that explores a single intervention time and location in advance, and develops it into a feedback control method such as Model Predictive Control. We develop Model Predictive Control by using ensemble prediction to deal with computational acceleration methods or uncertainties that could be rare-limiting in weather control method.
Methods
We predict weather by adding perturbation to conditions of numerical weather prediction model such as atmospheric pressure, wind speed, temperature and humidity at different locations and time, and analyze induced accumulated precipitation. We formulate inverse problems and optimization problems using a perturbation model to predict rainfall perturbations from state perturbations and develop a method to calculate the state perturbations required to achieve the desired rainfall perturbations. We also develop a method to calculate intervention amounts required to achieve the necessary state perturbations and demonstrate the feasibility of implementing feedforward control that predetermines intervention in advance. In addition, we develop a feedback method (Model Predictive Control) to decide the amount of intervention according to the actual weather, which changes from time to time. We integrate intervention calculation methods and data assimilation techniques and develop less computationally demanding methods to demonstrate the possibility of heavy rainfall mitigation over land by numerical simulations based on practical computation time and intervention volume.
At the start of the research, no specific numerical targets will be established, because the scale of heavy rainfall over the sea and the reduction in rainfall over land for disaster mitigation are expected to vary depending on the specific disaster events. By the FY 2024, we select cases with high potential to generate heavy rainfall over the sea based on the results of Item 5-2. Flood inundation and economic damage estimates for the selected cases will be calculated by Item 8-1 and 8-2 to investigate the extent to which the heavy rainfall generation over the sea and rainfall reduction over land are required. Then, we set target values for the rainfall reduction to be achieved for each selected cases at an early stage, to serve as our goals.
The algorithms and technologies developed in this study will be integrated into the weather control system development being promoted in Item 4-1.
Importance
Techniques that can calculate when, where and what extent the atmospheric conditions should be altered to change the precipitation, and what types and what extent interventions are needed to induce the change, are essential to the development of the weather control method for mitigating rainfall over land. It is important to see the atmospheric conditions and since perfect predictions are difficult, the intervention volume should d be adjusted based on the actual conditions at each time. Therefore, successful weather control needs to establish real-time feedback control methods, which integrate intervention calculation methods and data assimilation techniques. Since weather prediction itself requires high computational demand and its optimization necessitates even more calculations, the development of computational reduction techniques is also essential to realize feedback control.
Expected problems and solutions
Due to the chaotic nature of weather, achieving high-precision prediction over long periods is challenging, building high-precision perturbation model over long periods is also considered to be challenging. However, short-term macroscopic perturbation models can be constructed because short-term macroscopic causal relationships, such as the influence of upwind humidity on downwind rainfall, have been recognized. Guidelines can be established on how far the future predictions and optimizations should be made in Model Predictive Control based on the effective time range of the perturbation models. Guidelines can also be established for selecting conditions and intervention methods where the effects of perturbations are easy to predict. It is not sure whether intervention volume can be calculated within realistic computation time, but by limiting the locations and time frames for intervention, computational demands can be reduced. In the end, a trade-off between computational demand and optimality is expected to be necessary.
Members
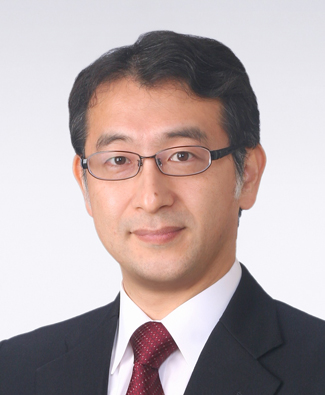
Item 1-2Development of data-driven control theory
Principal investigator: Masaki Ogura
Outline
This study develops weather control methods using data resources from large ensemble prediction based on meteorological observation information. Specifically, through comprehensive examination, adjustment, combination, and evaluation of existing data-driven robust control methods such as Model Predictive Control based on ensemble prediction, we develop data-driven robust control methods suitable for large-scale dynamic systems like weather phenomena with uncertainties in initial values and mathematical models.
Methods
Among the numerous existing data-driven control methods, we focus on Model Predictive Control methods utilizing methodologies such as particle filters, Monte Carlo methods, and deep unfolding, which are expected to have scalability required in weather control and compatibility with ensemble prediction. In addition, we ensure the diversity and sufficiency of the existing methods under examination by considering heuristic methods that do not have an established mathematical foundation. By reinterpreting these control methods from the perspective of weather control and making adjustments and integrations as necessary, we develop data-driven robust control methods suitable for weather phenomena.
At the start of the research, no specific numerical targets will be established, because the scale of heavy rainfall over the sea and the reduction in rainfall over land for disaster mitigation are expected to vary depending on the specific disaster events. By the FY 2024, we select cases with high potential to generate heavy rainfall over the sea based on the results of Item 5-2. Flood inundation and economic damage estimates for the selected cases will be calculated by Item 8-1 and 8-2 to investigate the extent to which the heavy rainfall generation over the sea and rainfall reduction over land are required. Then, we set target values for the rainfall reduction to be achieved for each selected cases at an early stage, to serve as our goals.
The algorithms and technologies developed in this study will be integrated into the weather control system development being promoted in Item 4-1.
Importance
We believe that achieving the goals of this project, “maritime heavy rainfall generation and rainfall damage reduction over land”, would be difficult without utilizing mathematical weather models and large ensemble prediction. We develop and evaluate methods for using large ensemble prediction, thereby demonstrating the feasibility of interventions on a computer. This is essential for the success of the project. In addition, technological outcomes of this study are expected to contribute not only to maritime heavy rainfall generation and rain fall damage reduction, but also to various weather control scenarios in the long term. Thus, they are expected to contribute to the overall Moonshot goals.
Expected problems and solutions
The effectiveness of the aforementioned data-driven control methods has already been confirmed for dynamic systems with relatively low-dimensional state spaces like tens or hundreds. We qualitatively assess that these methods can also be applied to large-scale systems with appropriate adjustment and modification. However, when advancing towards the actual application of these methods to mathematical weather models, which are huge dynamic systems, it is expected to be challenging in terms of computational volume. This challenge will be solved by starting with simpler models, such as the Lorenz model, and gradually scaling up the models while continuously doing research and development. Additionally, we actively incorporate computational efficiency techniques, such as those for accelerating learning, which have seen significant advancements in the field of artificial intelligence.
Members
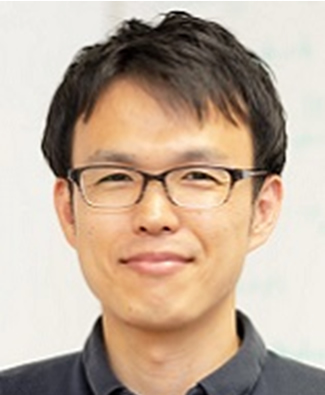