Quantifying Weather Controllability
Data from ensemble weather forecasts can be mapped to low-dimensional space using machine learning. Through the analysis of low-dimensional space, we aim to quantify weather controllability by searching for disaster/non-disaster separatrix that can avoid disaster with small operations.
Assume the situation in which a ball rolls through the landscape shown on the left.
A ball is rolling from the Initial Stage(top) to the front(bottom).
At the foot of the first mountain, the ball continues to roll either left or right. If the ball goes left, a large amount of energy is required in order for the yellow-green ball to cross the separatrix and reach the valley on the other side, because it must go up over a high ridge.
On the other hand, at the foot of the first mountain (at the “control input” in the figure), a small amount of energy can change the direction of the ball. In short, if you can find the foot of the first mountain where the separatrix starts, you can make a large difference with a small amount of energy.
The primary goal of the Mathematical Research Team is to draw such landscapes from actual meteorological phenomena such as typhoons. In addition, the team tries to identify practical control input for weather, such as at a fork in the road (at the foot of the first mountain in the left figure) where a course of meteorological phenomena can be changed to the desired direction by a small amount of energy through the predicting of weather phenomena.
Mathematical Research Team
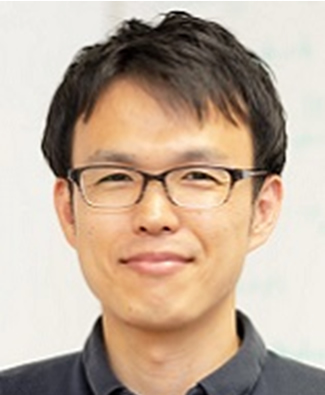
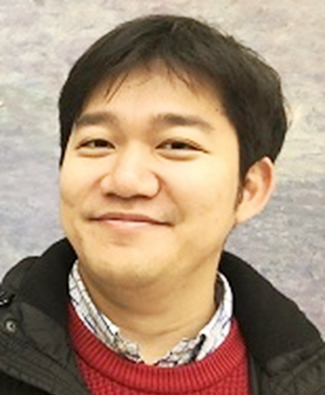
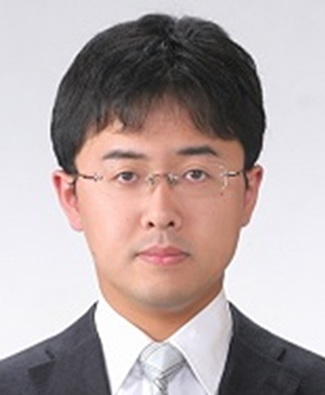
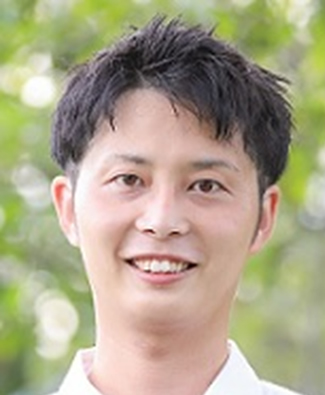
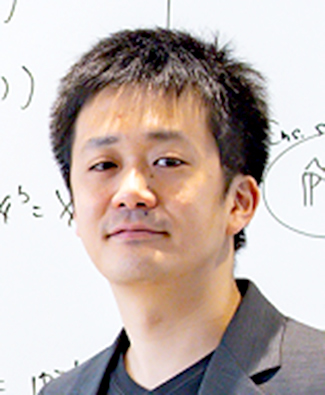
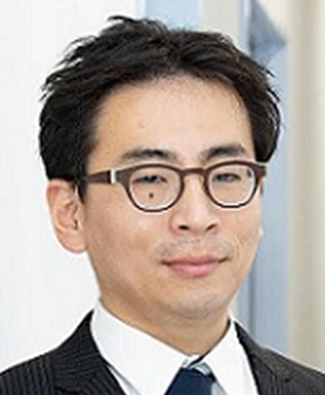
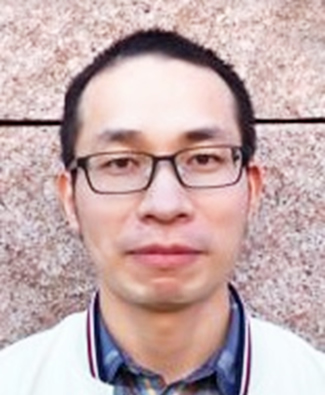
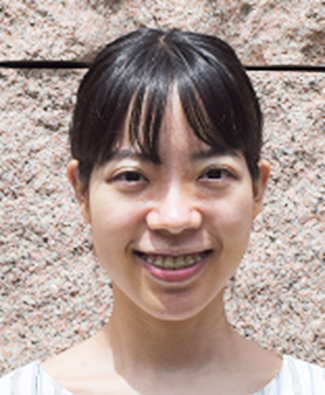
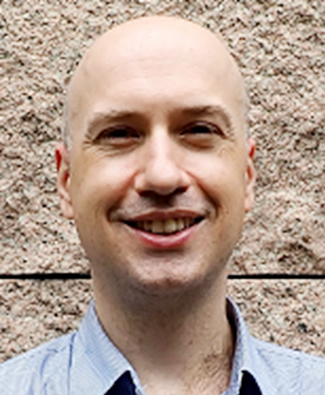
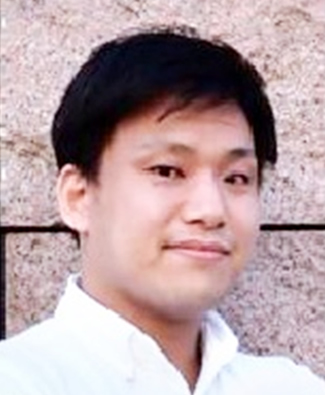
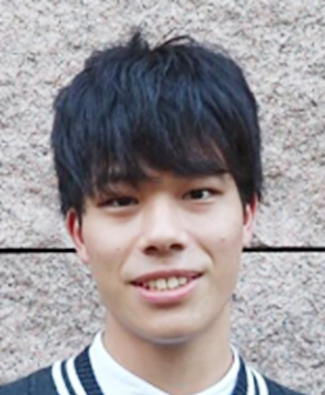
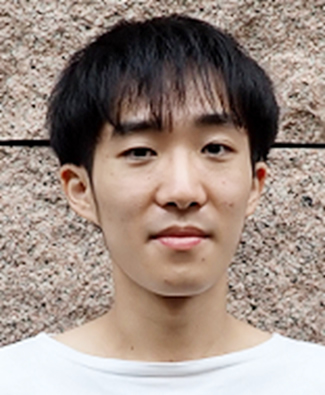
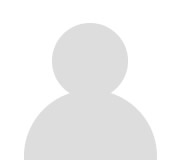
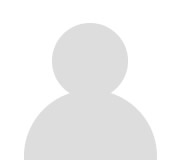